Welcome to the 2019 `Predict the Goose‘ forecasting competition taking place as part of ECON 345 ‘Applied Econometrics’ at UVic.
The Aim:
Predict the daily number of cyclists passing by the Galloping Goose Counter. Anyone is encouraged to enter the competition and submit a forecast!
The Rules:
Using the data provided here (download), create forecasts of daily cyclists counts. The data ends on Nov 10th, 2019. The period to be forecast is: 17 days from Nov 10th to Nov 26th 2019 (inclusive). The period that will be scored for forecast accuracy is Nov 20th-Nov 26th 2019.
Point forecasts of the number of cyclists should be submitted as a csv file, with the first column denoting the day (in format: dd/mm/yyyy), and the second column denoting the predicted number of cyclists as shown in the Table below:
date | cyclists | name |
---|---|---|
10/11/2019 | 312 | FirstName_LastName |
11/11/2019 | 364 | |
... | ... | |
26/11/2019 | 416 |
The csv file should be emailed to: fpretis@uvic.ca by November 20th, 7pm to be entered into the competition. Any form of prediction is allowed (empirical modelling using outside information, guessing, or even crystal balls). Just don’t go cycling by the counter to manipulate the numbers!
Scoring:
Forecasts will be scored using the root mean square error of the submitted point forecasts (density forecasts may be considered in future iterations of the competition). Results will be made available and prizes will be awarded end of November.
Previous Competitions
The Winners 2018:
Congratulations to UVic economics students Bryce Edwards & Mathieu Weary as well as Dr. Martin Farnham on winning the first `Predict the Goose’ competition! The competition invited students and faculty to forecast the number of daily cyclists on the Victoria Galloping Goose trail more than two weeks into the future and was organised as part of the UVic’s graduate time series econometrics course taught by Dr Felix Pretis. The winning entries used methods based on historical averages combined with expert judgment, exponential smoothing, as well as dynamic models taking weather forecasts into account. The winning entries accurately predicted the thousands of cyclists using the trail each day, with an average forecast error of only 15%.
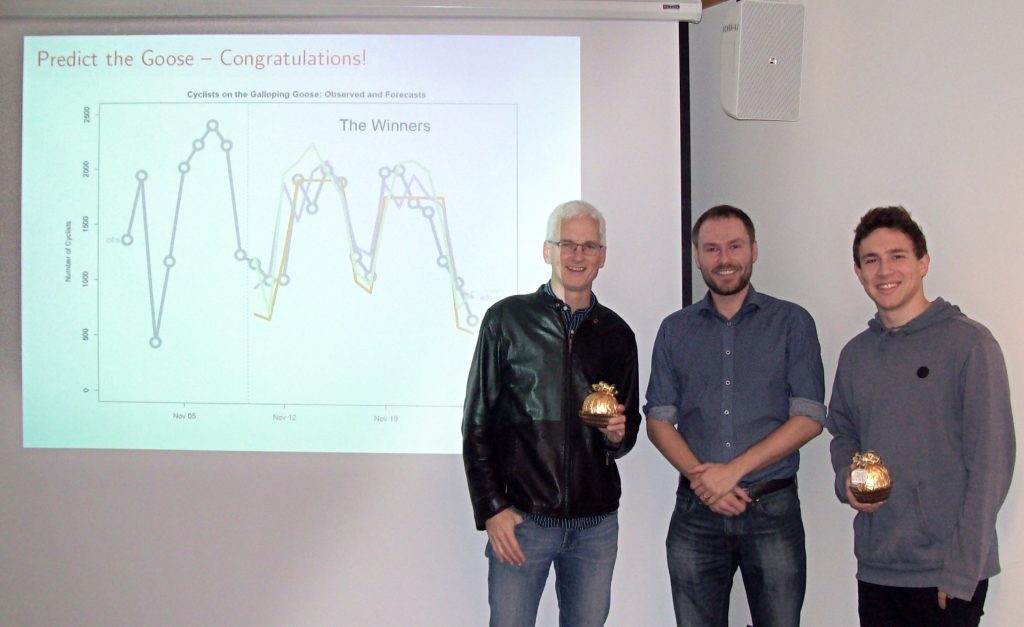
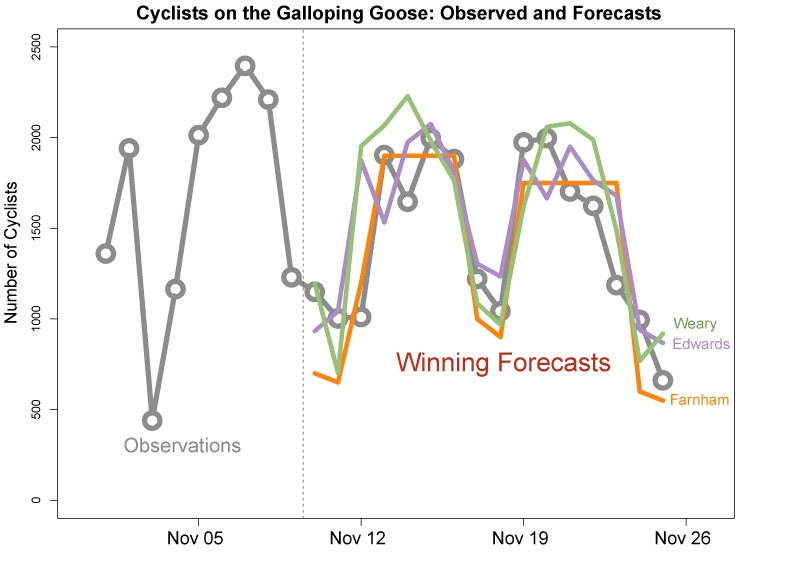